ML Teams Up with Marketers
Machine Learning can now be the key element in a Customer Relationship Management (CRM) tool to boost customer acquisition. American Express, a company that once received leads only via email campaigns, is now witnessing a 40 per cent increase through machine learning (ML)-driven online engagement. According to a Forrester report, ML technologies are expected to hit […]
Topics
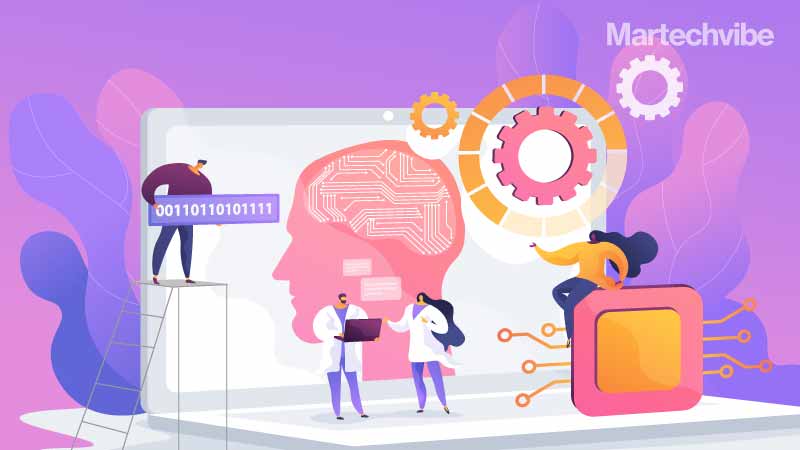
Machine Learning can now be the key element in a Customer Relationship Management (CRM) tool to boost customer acquisition.
American Express, a company that once received leads only via email campaigns, is now witnessing a 40 per cent increase through machine learning (ML)-driven online engagement. According to a Forrester report, ML technologies are expected to hit the $100 billion market by 2025. Specifically, ML as a Service (MLaaS) could hit over the $7 billion mark by 2023.
Organisations worldwide are realising that a Customer Relationship Management (CRM) tool that works with ML is the ideal plan for customer acquisition. While Gartner indicates that marketing automation is a popular sub-segment of CRM that has increased by over 18 per cent, it is being further improved today. Along with the usual features of a CRM, the addition of ML can upgrade the tool with the possibility of predictive analytics, improved automation of email marketing campaigns, and help with customer transactions.
For instance, ML analytics can create sample customers that can help marketers make persona-specific strategies. It gets easier to target specific customers when the company already possesses valid data points from “lookalikes”. Also, why send one blanket marketing collateral and wait for leads? Focused content creation is possible with data insights. It can assist marketers in figuring out the type of content that gets the most engagement and further create similar content. Pinterest acquired an ML-based company Kosei in 2015 for content discovery. Today, ML exists in every aspect of Pinterest’s business operations from content discovery and reducing churn rate to customer acquisition.
ML can help brands generate targeted leads. Research reveals that organisations that leveraged ML for lead generation witnessed a 50 per cent increase. Meanwhile, brands claim that data entry into their CRM system is a time-consuming task, and sometimes, it gets done ineffectively. By utilising ML for data collection, it became easier to use the already loaded customer information, purchase history and communication tracklist to create better marketing strategies. Additionally, customer service skyrockets with a quick response time, which can increase the chances of a repeat purchase and pave way for brand loyalty.
Exploring Segments of Acquisition with ML
While Amazon uses ML to enhance their recommendation systems, other brands leverage ML to reduce the cost of customer acquisition. Previously, customer segmentation was done manually by human resources and it was time-consuming. Today, ML helps marketers understand customer behaviour and create targeted content. Consider the fitness brand Under Armour. They use ML in their app to identify user segments and create customised fitness recommendations for prospective customers. Marketers want their data-based product recommendations to reach browsing users at the right time in any channel. ML makes that happen.
Social listening is another segment powered by ML. With ML-driven social listening tools, brands are able to keep track of brand mentions, hashtags and relatable content across social media channels. With such social activity insights, marketers can create content that attracts their audience. They can also obtain information from various other digital touchpoints and in-store visits to determine customer behaviour. The airline industry benefits the most out of ML-driven listening as flyers frequently take to Twitter to complain about their travel experiences. It also gets easier to keep track of competition and tailoring marketing campaigns and discounts based on the competitor’s shortcomings. Many times, customer acquisition through competition tracking via ML works effectively.
Understanding the Limitations
ML technology does not hold the power to solve all the complications that the customer acquisition process entails. It is important to continuously monitor the tools and provide support with further investments and partner with the most suitable vendors.
Global enterprises have the budget to invest in ML tools but SMBs continue to struggle in an extremely competitive market. Technology experts are looking to create affordable ML solutions for customer acquisition that can work well with legacy marketing systems as well. Many SMBs are waiting for the mass production of a cheaper model to hit the market.
On the other hand, there are some SMBs that altered their business budgets to occupy a heavy ML investment. For instance, the Serbian bank Raiffeisenbank invested in an ML-driven marketing campaign for a credit product. The ML solution was used on historical data, risk scores, and bill payment details to target people with a credit offer. The bank generated a 14 per cent success rate compared to their previous success rate of one per cent.
What does the future hold?
There will soon be a time when marketers will be able to derive insights from the shortest of marketing data that may otherwise seem insignificant. For instance, the subject line of emails sent to prospective leads. With insights on open rate, marketers can moderate their content.
The use of commercial ML in digital marketing is playing a major role in decreasing expenditure and resources. While the ML market is itself growing exponentially at a CAGR of 39 per cent, industries are expecting better solutions for marketers to garner leads.
Currently, the technology is used in several aspects of marketing including customer service, customer experience, and content. Experts believe that ML for customer acquisition is most visible in organisations that make a majority of their decisions based on data insights. From A/B testing marketing campaigns, content, and smart flow forms, brands have a higher chance of customer acquisition with ML.