Explained: Recommendation Engines
TLDR: Recommendation engines can help analyse and predict whether a particular user would prefer a product or not, based on the particular user’s profile and its historical information. Using ML algorithms, a recommendation engine has also proved to improve the business decision making processes and quality. Businesses now are seeking different ways to connect with […]
Topics
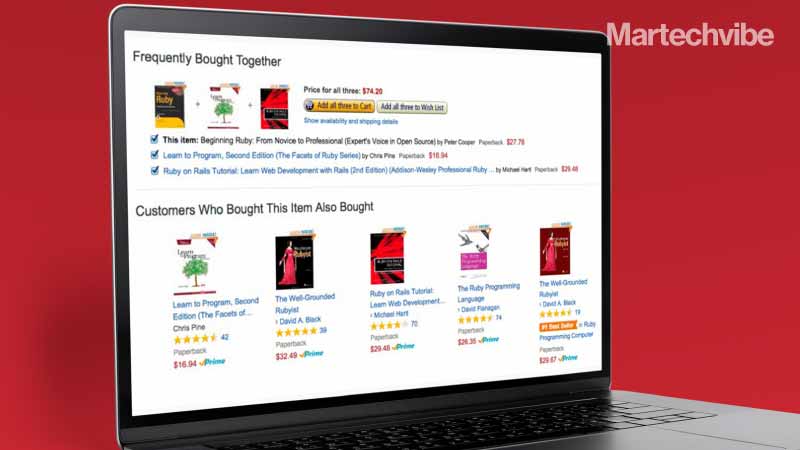
TLDR: Recommendation engines can help analyse and predict whether a particular user would prefer a product or not, based on the particular user’s profile and its historical information. Using ML algorithms, a recommendation engine has also proved to improve the business decision making processes and quality.
Businesses now are seeking different ways to connect with their customers, maximise value and increase engagement. Many have harnessed the power of machine learning (ML) and artificial intelligence to do so.
Be it eCommerce or traditional offline business, ML applications have come across as important. Furthermore, with a wide range of product and price options available, the need to filter, prioritise and efficiently deliver relevant information has increased over time. Enter recommendation engines.
How It Works
Recommendation engines help businesses gain valuable insights hidden within massive data. A fact-based method that contrasts traditional marketing, which generally relies on intuition, provides businesses with solutions that are not just mere assumptions. Recommendation engines can help analyse and predict whether a particular user would prefer a product or not, based on the particular user’s profile and its historical information. Using ML algorithms, a recommendation engine has also proved to improve the business decision making processes and quality.
Netflix uses a recommendation engine to present their viewers with enhanced movie and show suggestions, retail giants like Amazon, on the other hand, uses a recommendation engine to offer its customers with better product recommendations. Although every sector uses the technology for different purposes, all have the same goal: to drive sales, boost engagement and customer retention, and deliver more personalised customer experiences. Implementing such technology within the fabric enables companies to gain new customers, by sending out customised emails with links to new offers that meet the recipients’ interests, or suggestions of products and offerings that suit their particular profiles.
Types of Recommendation Engines
There are two main types of recommendation engines – collaborative filtering and content-based filtering.
The collaborative filtering method for recommender engines makes use of past interactions that have been recorded between users and items they engaged with to produce new recommendations. The collaborative filtering method also tends to find what similar users would like and the type of recommendations to be provided and to classify the users into similar clusters and recommend each user according to the preference of the cluster. This method is one of the most frequently used approaches and can be found in the recommendation engines of Youtube, Amazon, and Spotify.
Similarly, the content-based approach uses additional information about users or item features to recommend other items similar to what the particular user likes, based on their previous actions or feedback stored as data. Such a model helps in making new predictions for a user more easily, with a short analysis of the user profile, based on its information, determining relevant product items to suggest. The content-based recommendation suits best entertainment platforms like Netflix.
How Recommendation Engines Can Benefit Retail
A recommendation engine can help raise awareness of the brand or its new products among customers and increase revenue, customer satisfaction, and experience in several ways. Using tailored product recommendations can not only aid in generating higher click-through rates but also increase average order value and boost conversion rates. Intelligent product recommendations allow for more natural, logical opportunities to upsell and in turn, cross-sell to customers, automating offers suggestions through data. Small transactions can be turned into larger ones, and customers who might not have been browsing through the interface to make a purchase might suddenly find themselves with a full cart.
Studies suggest that up to 31 per cent of eCommerce site revenues today are generated from personalised product recommendations. Using a recommendation engine also provides a competitive advantage over brands that have not yet embraced personalisation and AI.
What’s Next?
With the constantly changing state of Big data and methods of data collection, the requirement for a more AI recommendation engine seems imperative. Incorporating time into a recommender system is a critical aspect due to the seasonal preference effects. Technologies such as recurrent neural networks, a powerful and robust neural network with an internal memory that can help the algorithm remember important elements from data, and deep learning are now being used by retail conglomerates to fix the problem.
If you liked reading this, you might like our other stories
How Spotify Has You Humming
Perform or Perish-Performance Marketing Trends