Tapping into the Subconscious Mind of the Consumer
Harvard Business School professor Gerald Zaltman says that 95 per cent of our purchase decision making takes place in the subconscious mind. It seems then that it’s a common misconception by most customers who believe that their buying decisions result from a well-thought-out rational analysis of available alternatives in the market. If Zaltman is to […]
Topics
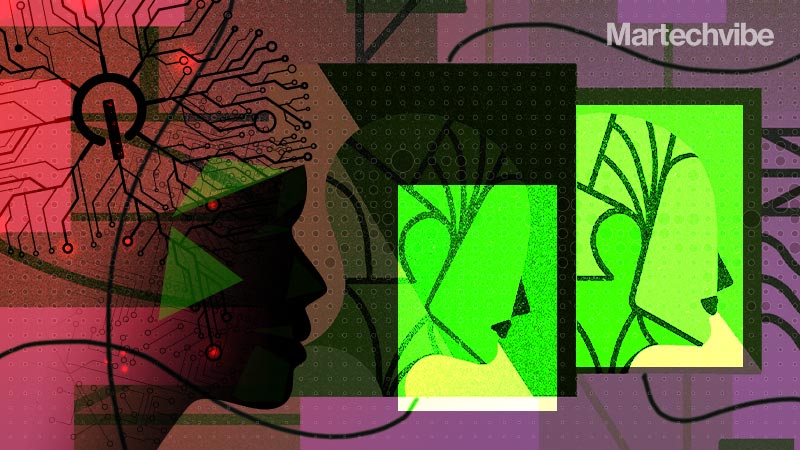
Harvard Business School professor Gerald Zaltman says that 95 per cent of our purchase decision making takes place in the subconscious mind.
It seems then that it’s a common misconception by most customers who believe that their buying decisions result from a well-thought-out rational analysis of available alternatives in the market. If Zaltman is to be believed, emotions decide how we buy.
Emotion AI uses artificial intelligence to study non-verbal cues of humans like body language, facial expressions, gestures, and tonality of voice to detect their emotional state.
Advanced algorithms detect and analyse the mood, attitude and emotional state of a consumer based on the position and movement of their facial muscles. At this time, software can draw out a score based on a variety of emotions like happiness, sadness, fear, disgust, anger, surprise or neutral.
The use of emotion AI at the point of sales presents a particular opportunity to make up for bad experiences, treat happy customers to convert them into loyal ones that will act as brand ambassadors and identify whom to re-engage with.
For POS systems, emotion AI leverages A/B testing of planograms to identify the one with the highest stopping, holding and sale closing power. A planogram is a diagram that shows how and where specific retail products should be placed on retail shelves or displays in order to increase customer purchases.
Planograms, coupled with emotion AI, provides insights on market shares per brand, impact on product sales, fitting the brand image and purchase intent. With retailers experimenting with digital and in-store blends, emotion AI-enabled can optimise virtual fitting rooms, or AR/VR-led in-store shopping experiences.
It is a quick way to identify the change of emotions or mood during different stages of customer interaction — before, during and after the interaction with the brand. For example, EnableX has a FaceAI API that intelligently analyses and measures facial expressions and emotions of one or multiple faces in real-time to deliver more natural, contextual and meaningful engagement experiences. It uses deep neural networks and human perception AI to analyse complex and dynamic human expressions in real-time.
Also known as affective computing, the term was first coined in 1995 by Rosalind Picard’s paper of the same name, published by the MIT Press. Biometric sensors, voice analysis, text analysis and computer vision are used for data collection.
Global Vox Populi, a market research, analytics and consulting firm uses eye tracking and facial coding to test package design through the eyes of the customers while they consider the items on the shelf. While these initial emotions are processed below the conscious level, facial coding assesses them, by measuring the emotional response to advertising, as manifested via changes in facial expression.
These measures are converted into an overall indication of positive, negative and neutral emotion from second to second during an ad, and an indication of which specific emotions are being felt.
Entropik Tech’s Affect Lab has a facial coding solution that claims to have an accuracy of more than 90 per cent in identifying all these emotions by just using a webcam/mobile cam. Facial emotion recognition is one of the most popular applications of emotion AI, with computer vision-based facial coding being the most scalable one. This technique is based on Paul Ekman’s Facial Action Coding System (FACS)4, a system used to classify facial expressions and movements as universally accepted emotions. FACS breaks down facial micro-expressions into individual components of muscle movement, called Action Units (AUs) and there are 7 emotion-related facial actions classified into 7 universal emotions: happiness, sadness, surprise, fear, anger, disgust and contempt. Machine learning algorithms are trained with each emotion separately to increase the precision and allow them to detect multiple emotions on the same face.
Nothing can replace the human touch of a clued in salesperson but emotion AI attempts to use technology to scale up the possibility of adding an affective message to an otherwise machine-led approach. The range of emotions is wide which means marketers are some time away from accurately identifying the complex scale.
If you liked reading this, you might like our other stories
Is Empathy The New Marketing Secret Sauce?
Emotions Make the Experience