Explained: Knowledge Discovery In Database
TLDR: Marketing involves digging into large datasets, locating anomalies and finding patterns to make informed business decision-making. Hence, it’s vital to understand how Knowledge Discovery in Database (KDD) can be an effective tool for marketers to make intelligent business decisions. Known as the happiest place on the Earth, Disney’s Animal Kingdom is the world’s largest […]
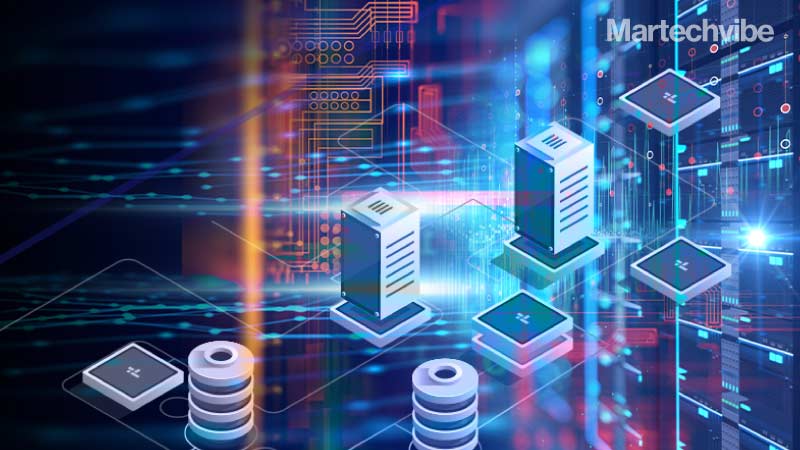
TLDR: Marketing involves digging into large datasets, locating anomalies and finding patterns to make informed business decision-making. Hence, it’s vital to understand how Knowledge Discovery in Database (KDD) can be an effective tool for marketers to make intelligent business decisions.
Known as the happiest place on the Earth, Disney’s Animal Kingdom is the world’s largest theme park – but do you know what is at the core of its design? Data. The world’s largest furniture brand IKEA has data at the centre of its digital strategy. The American airline JetBlue Airways handles all critical systems, from airport operations to customer experience, with data at its base. What do these examples tell us? Simply, the significance of data.
Organisations, small or large, are constantly being poured with data about consumer preferences. But, having a massive volume of data does not always lead to wiser choices. There’s a pain point here – the difficulty lies in extracting the essence of information from the available datasets to generate reports, views or data summaries for enhanced decision-making.
Today, marketers, in particular, are under intense pressure to utilise analytics to justify and inform their decisions. This is where Knowledge Discovery in Database (KDD) can ease the process. So, it’s essential to understand what KDD is.
The process
To start with, KDD is the non-trivial process of identifying valid, useful, novel and understandable patterns within datasets to derive valuable insights and consequently make impactful decisions. The process starts with determining the objectives of KDD and ends with implementing the discovered knowledge. Let’s understand the journey in detail.
Understanding the domain: In the first step, the people in charge of a KDD project need to comprehend and understand the user’s end goals and where the knowledge discovery process will take place. Additionally, building and grasping the application domain and acquiring relevant prior knowledge are essential.
Creating target dataset: After setting the objectives, the next step calls for identifying the set of data using neural networks, decision trees, clustering, etc., that will be used for the knowledge discovery process. The aspects that will be taken into account for the procedure include determining what data is accessible, getting crucial data, and then integrating all the data for knowledge discovery into one set. Simply put, the marketers need to:
- select (query) the dataset,
- identify subset variables (data attributes), and
- create data samples for the KDP
Cleaning of data: Data that needs to go as input in the model needs to be reliable; hence, it needs to be cleaned. The step aims to fix or remove corrupted, noisy, incorrectly formatted, duplicated, or irrelevant data from the target datasets and add missing values. This includes various statistical techniques or the use of a data mining algorithm.
Data transformation: Here, raw data sources are converted into a validated and ready-to-use format. The data transformation process is also referred to as ETL (extract, transform, load). The extraction phase involves identifying and pulling data from the numerous source systems where it’s created. The data is then moved to a single repository.
Model building and pattern mining: Data scientists might look at any intriguing data linkages, such as sequential patterns, association rules, or correlations, depending on the kind of study they are performing. It further requires using data visualisation and summarisation to present the pattern, or make data understandable, such that it is comprehensible to those from a non-technical background as well.
Lastly, knowledge representation: This technique utilises different visualisation tools to represent data mining results in the form of reports and tables and generate discriminant rules, characterisation rules, classification rules, etc., and the process starts over again.
The next thing to consider is the benefits of KDD and why it makes a perfect fit for marketers.
A helping hand
A marketer is responsible for monitoring market trends, preparing data-based strategies, creating advertising campaigns, developing pricing strategies and working with the company to create awareness of their offerings among consumers.
Three critical dimensions KDD provides to help marketers out include:
- Descriptive modelling: It unearths commonalities or clusters in historical data, such as consumer sentiment or product preferences, to analyse the reasons behind a campaign’s success or failure.
- Predictive modelling: The modelling helps identify future events or outcomes, such as credit scores, to predict customers’ ability to pay loans. It can uncover insights into campaign response, customer churn, and campaign or credit defaults.
- Prescriptive modelling: Text mining is the capability to effectively parse, transform and filter unstructured data, to include it in predictive models for enhanced prediction accuracy in light of the growth in unstructured data from the web, comment areas, books, emails, PDFs, audio, and other text sources.
Staying up-to-date with client behaviour, actions, and needs is crucial for marketers in today’s hyper-connected digital environment. With the use of knowledge discovery, they can aid businesses in predicting future market trends and conditions accurately. This helps companies stay relevant in a constantly changing world and offers them an advantage over their competitors. Further, being an iterative process, it only improves over time, meaning that new information gained during the process is passed back through it repeatedly, refining its quality each time.
Improved insights and strategies
Multiple challenges exist in marketing as data is highly unstructured, complex, and ill-defined. With KDD, marketers can dig into large customer databases that contain hidden customer information, which can be used to improve customer experience, enhance marketing initiatives, and forecast sales. By using precise data models, retail businesses can provide more focused marketing and improved offers to cater to customers more effectively.
If you liked reading this, you might like our other stories
Can Intent Driven Chatbots Enhance Your Business?
Martechvibe Explains: Identity Data