Are You Using AI For Dynamic Pricing?
AI can make dynamic pricing easier and better, as it facilitates continuous prediction, allowing for pivots for events in real-time Expense coverage and achieving a specific level of revenue criteria are critical for a business to grow and thrive. But how do you determine the right price for your goods and services? Use static prices, […]
Topics
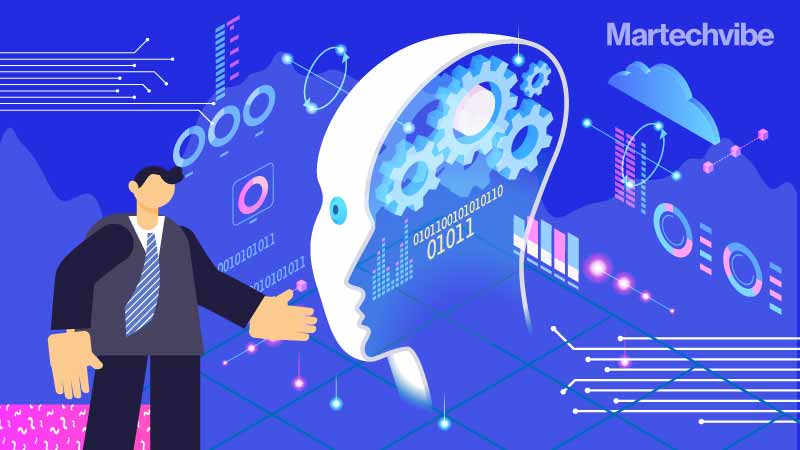
AI can make dynamic pricing easier and better, as it facilitates continuous prediction, allowing for pivots for events in real-time
Expense coverage and achieving a specific level of revenue criteria are critical for a business to grow and thrive. But how do you determine the right price for your goods and services? Use static prices, observe those of your competitors or a combination of both? The answer lies in setting a higher-level pricing strategy with the flexibility to make changes when needed.
Dynamic pricing is now being used by a range of industries – setting prices of product or services based on the state of market demands. With the tremendous amount of data generated by customers via surveys and social media, companies can use that data to adjust their pricing in real-time with dynamic pricing.
Processing the data allows companies to understand the current state of their market, make adjustments as needed, and maximise their opportunities to increase their revenue.
Amazon and Airbnb use this method to optimise their prices to attract more customers and increase profit. While traditional dynamic pricing algorithms use historical data to estimate the best prices, modern dynamic pricing algorithms leverage more data and AI and machine learning capabilities to predict market trends better and optimise prices accordingly.
Price optimisation and revenue management are two of the most prominent aspects that any business needs to address. However, inserting machine learning into the mix not only makes both tasks easier but efficient as well. Price optimisation with machine learning allows a company to have real-time pricing adjustments and efficiently respond to the marketplace to organise product campaigns accordingly and reach their goals.
The AI-Business Use Case
Pricing can be automated as required, either partly or entirely, depending on the goals of a product campaign. Modern algorithms also include analysing real-time data about competitors’ prices and stocks collected from websites using web scrapers or RPA bots.
In 2014, Amazon created a revolutionary algorithm, for which it was granted the predictive stocking patent. The system allowed retailers to reduce their delivery time and cost by simply predicting which customers would buy before they brought it. This allowed the retailers better control over pricing and their marketing strategy.
Businesses that actively respond to market demands develop better brand awareness with their pricing decisions and can inventory wisely with a better chance of survival despite the market conditions. Dynamic pricing helps a company increase its competitiveness and recover from a wrong move. For example, an airline can recuperate from a lack of sales during periods of low demand or just prior to an impending departure by having a sale on tickets for a particular flight or flights.
Dynamic pricing in eCommerce shifts away from standard static pricing, i.e. where items have a fixed cost, and uses data to price items instead. Therefore, the more data can be analysed, the better optimal prices can be shown. This means that pricing is no longer dependent only on how much stock there is. It uses a range of advanced analytics about the particular product, the buyer and competitors to determine the best price point to be served at any given time.
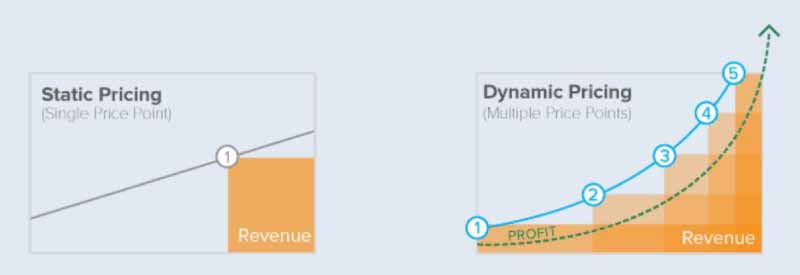
Image Source: Prisync
AI can make dynamic pricing easier and better, as it facilitates continuous prediction, allowing for pivots for events in real-time. At the same time, you can predict some of these events with historical data, not all.
With AI, one can identify happenings much faster and adjust the price within minutes instead of once per quarter. AI can review numbers more quickly than humans, taking them out of the loop for faster results. By using AI for dynamic pricing, an organisation can save people time, impact revenue, and aids market reaction time.
With the amount of big data gathered and analysed today, a lot can be inferred by glancing at a person’s browsing history and search patterns. The choice of a customer becomes a viable prediction. If executed cleverly, this can lead to a significant spike in profits, for example, pop-up ads you see on various sites showing things consumers find hard to resist.
Types and Key Considerations For Dynamic Pricing
Depending on the required mathematical model, businesses can create numerous algorithms that fit their dynamic pricing strategy:
- Bayesian model: The user picks a prior value indicating the initial belief about the possible price. Then, whenever a new data point is entered into the algorithm, the initial belief shifts either higher or lower. Most Bayesian models use historical pricing data as the most important feature to decide on the final price.
- Reinforcement learning model: An RL dynamic pricing model explores data about customers’ demand, considering seasonality, competitors’ prices, and the uncertainty of the market, with high revenue being the final goal.
- Decision tree model: Decision tree dynamic pricing algorithms help businesses understand which parameters have the most effect on the prices, and which of these price ranges predicts the highest revenues, and using this information, the algorithm predicts the best price range for each product.
To choose the best dynamic pricing algorithm, businesses need to take into account that the algorithm should be able to provide prices which:
- Maximise the profit
- Minimise customer churn
- Attract competitors’ customers
- Improve customers’ experience and maintain loyalty
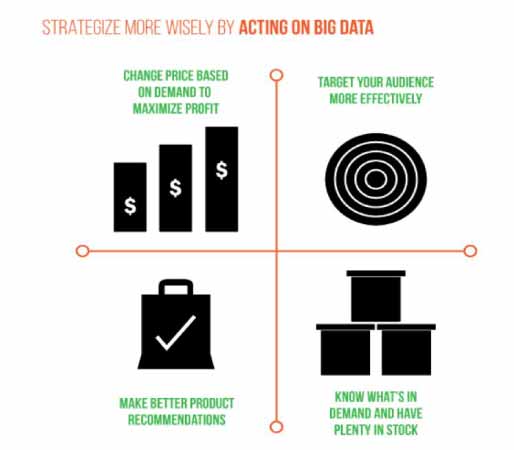
Image Source: Prisync
In actual use cases, increasing prices when you know your competitor’s stocks are running low. If they go to a website and find it unavailable, consumers who want the product right away will be more than likely to buy it from a store for a higher price, if they know they can get the item sooner.
When conversions are low, AI can be partnered with dynamic pricing to lower prices to increase conversions to a rate one is happy with.
AI identifies patterns in data to reveal pricing gaps in the market and shows opportunities missed, and when the AI software has identified these various patterns, algorithms based on the data can be customised to facilitate the price determination process to predict prices based on that information.
The benefit of doing this through AI is that it becomes automatic, flexible and completely scalable.
Past Backlashes
The flip side of dynamic pricing is that it can also create customer ire. Uber’s dynamic pricing, for instance, caused “several issues” when clients were charged preposterous fees for short rides due to extremely high demand. For instance, on New Year’s Eve, an Edmonton Uber customer Matt Lindsay was charged $1,114.71 for a 20-minute long ride that appeared in numerous newspapers.
Others also tend to complain about their bad experiences on the Internet despite being notified about surge rates via the app or being warned by drivers (the situation with Matt). Therefore, price transparency is one of today’s market traits, an environment where consumers can find which merchant provides an item or service of interest for a lower price in several clicks or taps.
Nevertheless, since extreme events like New Year’s Eve happen once a year, researchers have to deal with a lack of data to data sparsity. To solve this problem, companies are now using a custom LSTM (long short-term memory) model, an artificial recurrent neural network with the ability to remember information for long periods.
These models show good prediction results with time-series data containing observations taken at regular intervals. Such observations derived are usually numerical values.
The importance of an effective pricing strategy for running any business is hard to deny. At the same time, entrepreneurs can benefit from technological advances that come with increased computing speed, decreased data storage, and greater data availability for exploratory analysis to respond to changing market conditions with reasonable prices.
AI and machine learning now aid businesses in implementing dynamic pricing on a large scale while considering hundreds, if not thousands, of pricing factors, including price elasticity, and showing specific prices to customer segments with corresponding willingness to pay.
With the neck-breaking pace technology is developing, a business cannot survive without amalgamating it with its marketing strategy. It’s time for companies to step out of their comfort zones and use the online environment to its fullest, not just to predict prices but also to take care of their customers.
If you liked reading this, you might like our other stories
How AI Is Revolutionising B2B Pricing
Brands Commit to Increase Digital CX Investment: Report