AI-Augmented Open-Ends for Marketing, CX and Beyond
AI-augmented open-ends are revolutionising market research by combining the scale of quantitative surveys with the depth of qualitative insights — enabling faster analysis, richer feedback, and more strategic decision-making.
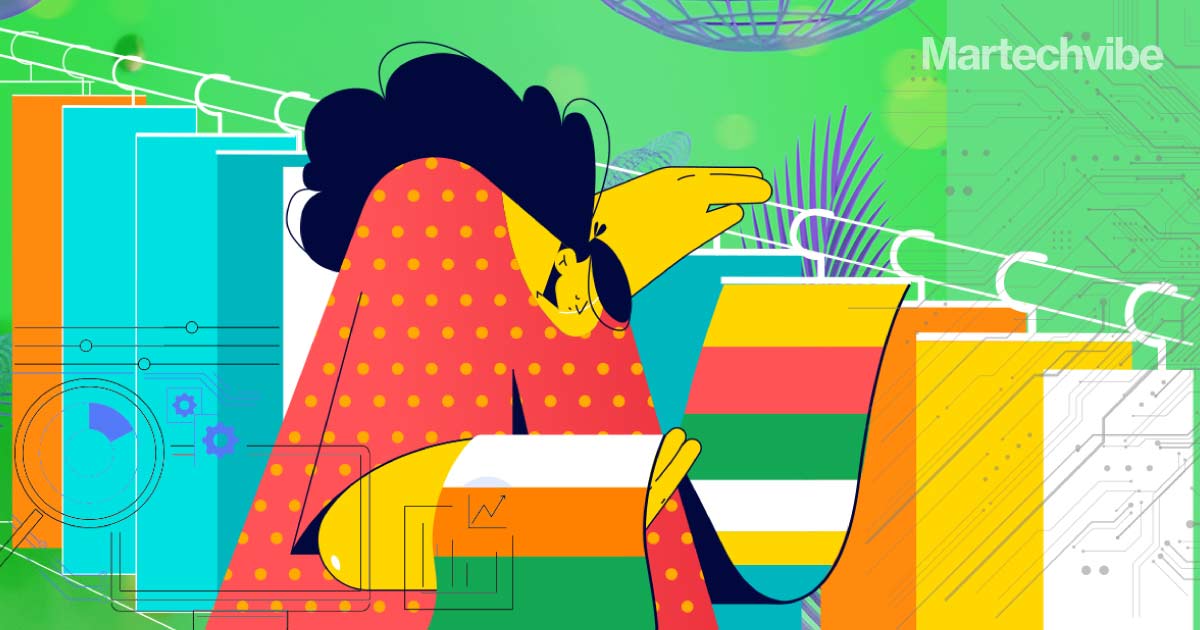
At the heart of effective market research lies the ability to genuinely understand consumer sentiment, uncover hidden motivations, and explain the “why” behind decision making. Traditional quantitative research techniques often rely heavily on structured surveys with limited scope for qualitative exploration. Conversely, purely qualitative methodologies can be challenging to scale.
Enter AI-augmented open-ends — a methodology that combines the scale of quantitative research with the depth of qualitative insights, powered by AI to unlock richer, more actionable understanding.
This novel approach is not only improving data quality, but is encouraging more detailed, descriptive feedback, and in turn, enhancing the depth and actionability of insights. By utilising AI-driven probing, researchers can dynamically generate follow-up questions and in-survey indicators based on initial responses, prompting respondents to elaborate further on their original feedback.
This poses another challenge for researchers – what do to with all of the unstructured data. Traditional open-end analysis is already a daunting task, but with AI integration, the volume, depth, and complexity of responses is even greater. Establishing an AI-assisted workflow is essential in structuring the unstructured, getting to insights quickly and without introducing unnecessary bias.
The core components driving successful adoption of AI-Augmented Open-Ends include:
- Seamless integration in the survey instrument. A pre-trained model providing probes and responses in-line with the rest of the survey drives a conversational feel, extracting valuable insights from respondents.
- Human led, AI-assisted code frame generation. Large Language Models (LLMs) are excellent at digesting and summarising key themes from unstructured data at scale, and with human guidance, are much more efficient than traditional manual processes.
- AI-assisted open-end coding. A hybrid approach where researchers provide project-specific training data to refine LLM outputs has shown to be indistinguishable from traditional human coding.
Once this workflow is established, researchers can transform traditional processes through AI-augmented open-ends, enhancing operations and activating new applications.
Three Key Applications Powered by AI-Augmented Open-Ends
1. Testing Business Hypotheses
One of the significant advantages of AI-augmented open-ends is the ability to effectively test business hypotheses. Historically, open-ended responses were overlooked in hypothesis testing due to analytical limitations and overall lack of depth. Now, advanced AI tools are able to extract more specific, detailed feedback, facilitate thematic extraction and automate coding, enabling researchers to quantitatively assess the presence and relevance of specific themes.
How AI-Augmented Open-Ends Accelerate Insight Generation:
A leading quick-service restaurant leveraged AI-augmented open-ends to evaluate customer-identified areas for improvement within a competitive product category. Through AI-generated follow-up questions, the restaurant significantly enriched response quality, capturing deeper and more nuanced insights. AI-driven coding and thematic extraction dramatically accelerated the analytic process from days to hours, reducing subjectivity and increasing consistency. Ultimately, this enabled the restaurant to rapidly validate some and deprioritise other hypotheses, directly informing product innovation and marketing strategies.
2. Simplifying Complex Qualitative Lines of Questioning
Complex qualitative pathing within quantitative studies traditionally involves rigid, predetermined question flows, leading to respondent fatigue and limited insights. AI-augmented open-ends disrupt this model by enabling more natural, conversational survey flows, significantly enhancing the respondent experience and, in turn, leading to better data quality.
How AI-Driven Probing Improved Data Integrity and Reduced Fatigue:
A healthcare provider had been using a series of 10+ open-ended questions at the end of a traditional quantitative study with mixed results. After integrating AI-augmented open-ends, this was reduced to 3 core questions with conversational follow-up components and AI-driven motivational probes. The transition to a more organic flow reduced fatigue due to shorter, more relevant lines of questioning, resulting in improved data integrity and more reliable qualitative insights.
3. Getting More out of Hard-to-Reach Audiences
The value of AI-augmented open-ends extends beyond scalability. Engaging niche and hard to reach audiences is costly and challenging, making it essential to maximise the value of each response. In the B2B space, AI-driven probing enhances the depth and quality of insights, empowering more informed business decision making.
How AI-Driven Probing Uncovered Hidden Customer Pain Points:
An automotive software company had been conducting a customer satisfaction study for 8+ years to better understand their customers’ pain points and enhance their services. Each year, the same open-ended question was asked: “Why are you dissatisfied with [product]?” However, they found that the responses only scratched the surface of customers’ true concerns. After introducing an AI-augmented open-end, they were able to extract deeper insights into the reasons behind customer dissatisfaction. As a result, they were able to identify and address issues they weren’t previously aware of, significantly improving their customer relationships.
ALSO READ: Is Sentient Marketing The Future of AI-Driven Customer Connection?
Best Practices for Implementation
While the impact of integrating AI-augmented open-ends into market research is profound, realising the benefits requires thoughtful implementation.
Best practices for successful implementation include:
- Balance AI with Human Oversight: While AI workflows significantly enhance efficiency and objectivity, human expertise is essential for aligning insights with business objectives.
- Optimise Respondent Experience: Leveraging AI to reduce respondent fatigue and improve engagement results in higher-quality data, however, the application must be intentional. Misuses or overuse of these tools can be cumbersome, and ultimately backfire on the core objective.
- Pre-train Models: AI-powered solutions are only as strong as the effort put in upfront. While out of the box models may seem like low hanging fruit for integration, the insights captured will be shallow and may not align with core research objectives. Pre-training models ensure results directly support strategic decisions.
What’s Next for AI in Market Research
AI’s influence on market research is just beginning. Future applications will further blend qualitative and quantitative methods through conversational data collection frameworks, making research participation more convenient for respondents and streamlining analytical workflows. Researchers and businesses that embrace these innovations will enhance their understanding of the market and drive more strategic, customer-focused decisions.
Ultimately, AI-augmented open-ends and AI-assisted workflows represent a significant leap forward in extracting qualitative insights through traditional quantitative channels, transforming the respondent experience, and analysing unstructured data at scale. By embracing these technologies, companies can unlock deeper, richer consumer narratives, fueling innovation and strategic growth.
ALSO READ: An Entrepreneurial Mindset Drives CX and Martech Success (and Fun)