Why Some Enterprises Consider Their Data Irrelevant
Trust is rarely about the deployment of new technologies but more to do with the way data is stored and managed across the enterprise.
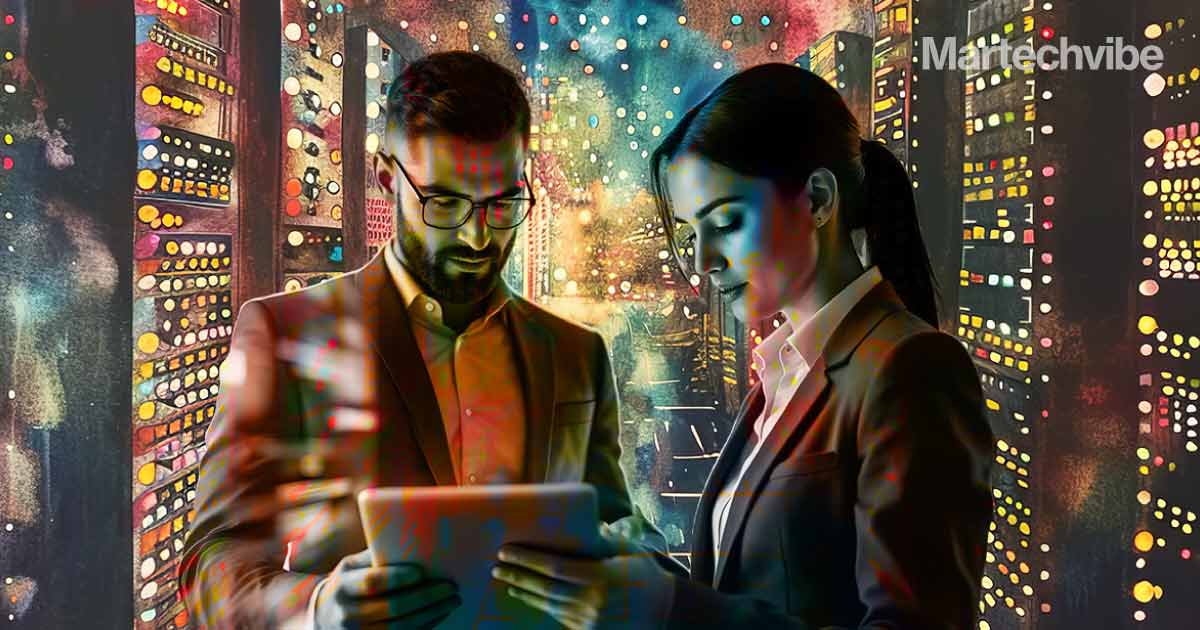
Data has a trust problem. While it is arguably an organisation’s most valuable asset, with data-driven companies 23 times more likely to acquire customers (according to the McKinsey Global Institute), the sheer volume of data now being generated has made it more difficult for decision-makers to place their trust in it. The output from data-powered processes, once sought after and hailed as invaluable, are now increasingly being met with scrutiny and scepticism due to a growing sense of “data irrelevance”. This crisis of confidence doesn’t just affect isolated pockets of a business; it spreads, stalling decision-making and hampering operational efficiency across the board.
At the heart of this issue lies a complex web of challenges: siloed data that creates fragmented perspectives; inconsistent metrics that confuse rather than clarify; and outdated information that no longer aligns with the current realities of the business. These problems contribute to a broader mistrust in AI outputs and the data that feeds into them, leaving operations leaders in a state of paralysis. As enterprises struggle to navigate this growing problem, the need to restore trust in data becomes not just a priority, but a necessity for survival in an increasingly competitive, data-hungry environment. Here are some of the biggest issues impacting businesses when it comes to trust in their data.
The Roots of Data Mistrust
Innovation isn’t linear, and technologies don’t evolve at the same pace. While operations leaders are quick to jump on new developments like Generative AI and AI-driven automation, their data – and the way they handle their data – is rarely ready for it. When new data rushes into the organisation, quite often it’s parked and siloed for use by a particular application. The more this process repeats itself, the more distorted and unwieldy the stockpile of data becomes. When data is confined to isolated departments or systems, it leads to fragmented views of operations. This fragmentation creates gaps in understanding, as different teams work with different pieces of the puzzle, often unaware of the full picture. These silos make it impossible to get a holistic view, making it difficult to draw accurate insights or make informed decisions. When operations leaders are forced to piece together fragmented data, they lose confidence in its accuracy and relevance, fueling doubt and undermining trust in the entire data ecosystem.
In many enterprises, different teams use varying definitions and metrics to measure similar outcomes, leading to confusion and conflicting interpretations of the same data. Without standardised practices, the same piece of data can be interpreted in multiple ways, depending on who is looking at it. In other words, “truth” becomes a fluid concept. This lack of consistency not only makes it challenging to compare and cross-reference data, but also erodes the credibility of the data itself. When combined with the use of outdated information, which fails to reflect the current state of the business, the result is a toxic mix that stops leaders in their tracks.
The Growing Effects of Decision Paralysis
When mistrust in data takes hold, one of the most immediate and damaging consequences is decision paralysis. Leaders who are uncertain about the reliability of their data are hesitant to make bold decisions, fearing that they may be acting on flawed or outdated information. This hesitation can lead to missed opportunities, as leaders struggle to respond quickly to market changes. The constant second-guessing and need for additional validation stifle decision-making processes, often causing companies to fall behind more agile competitors who can move with greater confidence.
It also leads to operational friction. Relying on poor-quality data forces teams to spend an inordinate amount of time verifying and cross-referencing information instead of focusing on the big picture. This not only reduces productivity but also increases the likelihood of errors. In some cases, the costs of these inefficiencies are tangible – such as lost revenue from delayed product launches or service improvements – while in others, the damage is more insidious, eroding team morale and fostering a culture of indecision. At this point, while not impossible, it’s very difficult to find a route back to a positive data culture.
Restoring Trust in Data
Trust is hard won. It’s rarely about the deployment of new technologies such as AI, but more to do with the way data is stored and managed across the enterprise. It isn’t the data itself that brands mistrust, but the way it is fragmented and classified, leading to contradictions and assumptions that begin to sow seeds of doubt. There are some key areas in which businesses should focus their efforts in order to avoid such scenarios:
- Breaking Down Silos
Data integration involves consolidating disparate sources into a single platform that is accessible to all relevant departments. This holistic approach ensures that everyone in the team is working from the same data set, reducing inconsistencies and providing a more accurate picture of operations. Advanced integration tools can facilitate this process by seamlessly merging data from various systems, allowing for real-time updates and continuous flow of information. When data is integrated effectively, it becomes a reliable asset rather than a source of confusion and doubt, and lays a strong foundation for new data-driven technologies and processes. - Achieving Consistency
Inconsistent metrics and definitions across departments are a major cause of data mistrust. By creating a common language for data across the entire company, businesses can ensure that all teams are aligned in their understanding and use of data. This consistency is crucial for making data more actionable, as it allows operations leaders to compare and analyse data output with confidence. Implementing clear, organisation-wide standards for metrics not only enhances the credibility of the data but also fosters greater collaboration and trust among teams. - Keeping Information Relevant
Outdated information is one of the biggest challenges in maintaining data relevance. To address this, enterprises should invest in systems that enable real-time data processing and continuous updates. These systems ensure that the data being used reflects the most current state of the business, allowing decision-makers to respond swiftly to changes in the market or internal operations. Real-time data not only enhances the accuracy of decisions, but inherently builds trust in the data itself because it eliminates the risk of encountering obsolete information.
Achieving these things isn’t easy, but the task can be broken down into achievable milestones. First, organisations must prioritise data governance by appointing dedicated teams to oversee the integration and standardisation processes. These teams should be responsible for ensuring that data is consistently cleaned, validated, and updated. Regular audits of data quality and adherence to standards can also help to maintain the integrity of the data over time. Beyond that, businesses should work hard to foster a culture of transparency and collaboration, with data shared securely across relevant departments. This avoids the “black box” scenario, where employees aren’t sure where the data has come from or how a decision has been made.
Data hasn’t lost its value, but the methods we need to employ in order to extract that value have changed. The goalposts haven’t moved, but the playing surface has expanded and tactics need to change. By reevaluating their approach to data and how it is handled, organisations can carry on using data to its fullest potential and restore their faith in the game.