Businesses Need to Focus on Uplifting Technique to Reduce Consumer Costs
While making a decision, it is important to consider the ROI and Uplift modelling is a technique that determines the possible gain by acquiring the resources to buy a product. Over the last few years, analytics and predictive models have encouraged progressive marketers to get ahead. And, as these techniques get more common, the competition […]
Topics
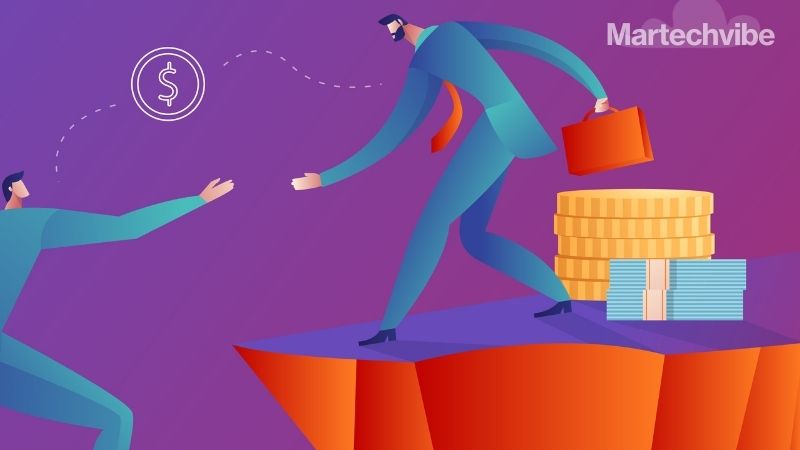
While making a decision, it is important to consider the ROI and Uplift modelling is a technique that determines the possible gain by acquiring the resources to buy a product.
Over the last few years, analytics and predictive models have encouraged progressive marketers to get ahead. And, as these techniques get more common, the competition will get tougher.
Uplift modelling is the next big edge for data-driven businesses. It focuses on the core of what matters to the businesses and consumers, responding to a marketing campaign. This is beneficial for brands to target the scarce resources from where they are likely to gain higher ROI and delivers marketing experiences that the customers want.
Understanding the utilisation of uplift models can benefit from supercharging the operations of marketing. Data scientists or vendors can use algorithms and below are a few strategies brands need to know about uplift models.
Move From Descriptive To Prescriptive Data
It is a prescriptive technique that informs brands about the actions to take to optimise their businesses, rather than just providing information. Demand and usage of predictive modelling are on the rise, as most businesses are analytical and technology is available. Uplift modelling works if a brand’s goal is not just to understand or predict the outcome, but also influence it, with data collection from descriptive analytics,
Also Read: How can Enterprises Avoid Social Media Marketing Pitfalls?
When Do Uplift Models Work Best?
Uplift modelling can be a radical shift for many organisations, it’s not a solution that can be implemented quickly. Building an uplift model requires first running an A/B test where some users are selected to receive the mediation that the brand is interested in testing. This extra data demand can strain resources, so it’s critical to know when the benefits are worth that extra effort.
Uplift modelling inclines to work well when:
The business goal is to impact interim user behaviour (a transaction). If the goal is to strengthen the consumer relationship or impact long-term metrics such as CLV, uplift may not be the best solution.
The brand has powerful marketing incentives at their clearance. If their intervention cannot master consumer behaviour, there won’t be any uplift for their model to find.
In the data science community, uplift modelling is still an area of research. However, its functional applications are rapidly gaining strength in the business world. Fidelity Investments, Uber and Wayfair are a few companies that hugely depend on uplift modelling.