Five Ways AI Can Enhance Product Discovery
AI uses advanced algorithms to read customer data and personalise product recommendations, thereby enhancing the product discovery process.
Topics
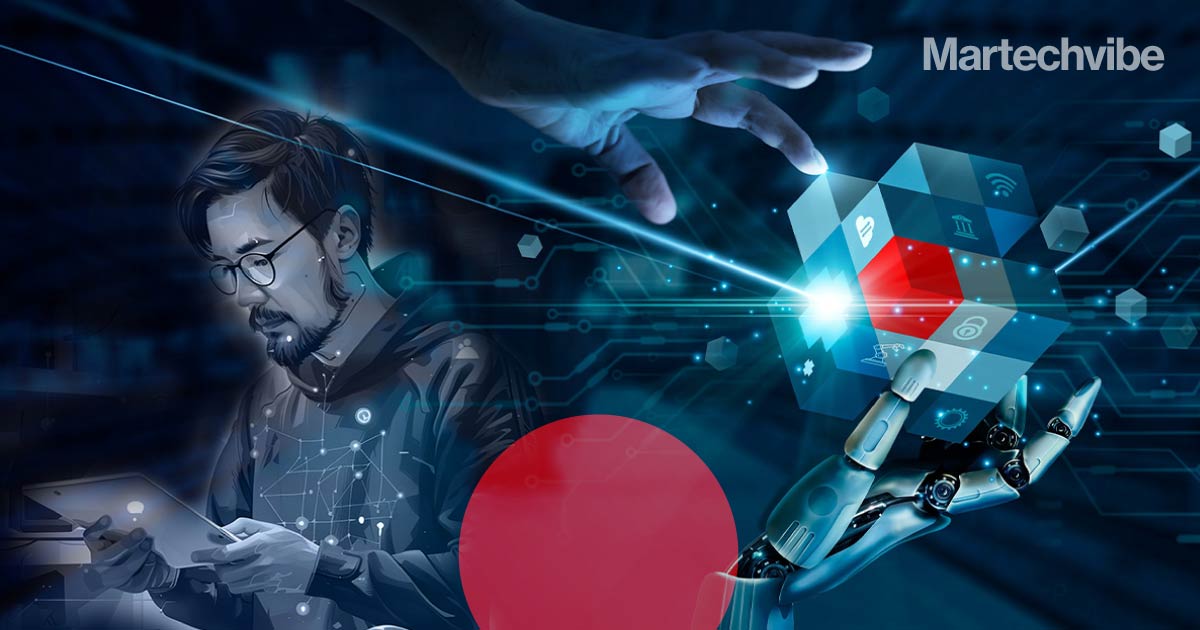
Ecommerce is fast evolving to suit customer needs. The younger generations that form the majority of businesses’ target markets, are adept at using the latest technology, and they expect the brands they shop from to incorporate the same.
The ecommerce marketplace is full of customer data and brands can leverage AI to quickly and accurately assess this information. Customers demand personalisation that grabs their attention and with the use of advanced algorithms, businesses can offer it successfully.
Artificial intelligence isn’t perfect yet as a technology. However, it is efficient in automating many manual tasks, thereby saving time and effort for human resources to focus on more complex requirements of the business. Here’s a look at how AI can understand customer data in the form of purchase history and buying behaviour to enhance product discovery for customers.
What is product discovery?
The process of product discovery entails customers being introduced to new products or other options that they would not have come across in their first search. The recommendations presented to customers originate from the work of AI models that leverage purchase behaviour, browsing history and patterns to offer personalisation. AI helps provide suggestions that are relevant and appealing to the customer, which helps in increasing the chances of purchase and gives the customer an enhanced shopping experience. Some customers also step forward to making impulse purchases.
Five Use Cases for AI in Product Discovery
Brands can find immense potential for AI in the product discovery process. Using advance machine learning algorithms that can read purchase patterns and behaviour, AI can assess massive customer data in no time and help tailor the customer experience by offering personalised and relevant product recommendations. Here are five use cases for artificial intelligence in product discovery;
Automated Translation and Localisation
Localisation is a crucial part of geography-based personalisation. Based on which country or region your customer is from, a brand must provide their content in the native languages. Translation that used to be a manual and resource-heavy task requiring both long hours and effort, can be automated now with genAI. Information like product descriptions and user ratings can be quickly translated to the user’s preferred language with the help of machine learning and large language models.
So, for a customer in China browsing a French fashion brand’s application, the content will be quickly translated to Mandarin, or any other Chinese dialect that the customer would prefer. This naturally helps brands expand their scope of user acquisition.
Comprehensive Product Descriptions
The ecommerce marketplace is much bigger than the average physical store. While maintaining inventory and keeping salespeople updated with product information is a daunting task as is in a physical store, it is an even bigger challenge when the store is online. The salesperson is replaced by the application and the content must be self-sufficient for every customer to get what they are looking for.
GenAI plays an important role here in automatically updating all necessary information throughout the application. Given the vast array of products listed on the application, AI can quickly identify any gaps in information across all categories, old and new, and use reference and comparison as a mechanism to fill all blanks. For instance, if a new line of shoes have been introduced and certain attributes are missing, genAI can come in handy for comparing previously listed lots of similar footwear to understand what all information is necessary, and update it without requiring human assistance.
Personalised Recommendations
It is well established that personalisation is a must for brands to have a competitive edge. However, personalisation at scale is a herculean task and machine learning algorithms can aid in customising the user journey. For example, customers often discover a brand through online ads they come across on social media. A customer might hop on to an ad attracted to a discount offer but if the app ends up showing generic products that don’t cater to the customer’s preferences, they might end up exiting. This leads directly to cart abandonment.
ML algorithms can utilise the customer’s purchase behaviour and browsing history to assess their preferences, and sort the products that appear on the app to suit the customer’s choices.
Also Read: This Week on the Grill: Amr Helmy, Red Bull, on Keeping Up With The Customer
Dynamic Suggestions
Customers can stay on a brand’s application for long periods of time browsing through items. Based on how a customer’s choices change, the suggestions offered by the brand must change as well. Machine learning algorithms, with the help of reinforcement learning, can learn about the customer’s changing activities and preferences, and modify and update the product suggestions and recommendations accordingly.
The algorithm can refine its recommendations using customers’ engagement metrics and purchase behaviour. This way, the process of product discovery becomes a cake walk for the customer, and if customers value one thing more than anything else, it is convenience.
Enhanced Search Process
The most widely used search engine is Google, and it is intelligent enough to catch errors like typos in search queries. The “Did you mean” feature by Google is powered by large language models (LLMs) that help detect what the user meant by what they typed. This means, algorithms are good at predicting user intent and improving search results based on that.
Today, if a customer lands on an application which fails to provide enough or accurate results simply because they mistyped a letter or two, it ends up in a demotivating customer experience. The brand will most likely lose this customer to a competitor that utilises advanced ML models to detect customer intent, thereby reducing the moments of frustration.
Lokesh Dhakar, Principal Product Manager at Grab, will take the stage at Unlocked: The Mobile & App Growth Summit, on 5 September 2024 in Singapore, to speak on “Product Discovery In The Era of Generative AI,” presenting case studies to explain how search and recommendations are evolving with the advancement of generative AI and LLMs.
Click here to register.