How Can Contextual Intelligence Elevate Healthcare and Retail
From personalised treatment plans to automating inventory management processes, with predictive insights, contextual intelligence (CI) can be the disruption brands need.
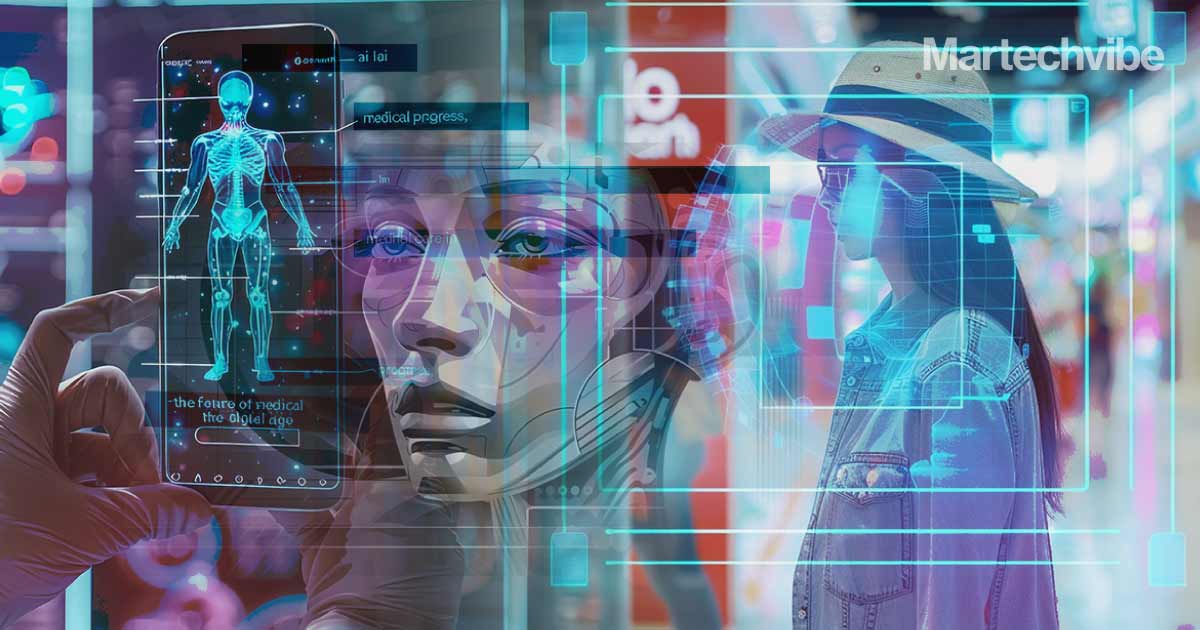
As of June 2024, genAI’s IQ is 155, the same as Elon Musk. For perspective, Albert Einstein had an IQ of 162. Just 7 IQ points higher, and you’re at the level of breaking down quantum science and understanding the universe. AI is doubling its intelligence every 5 to 6 months. By January 2025, AI will have an IQ of 310+ and 600+ by January 2026. The average human IQ is 80 to 110.
Implementation of Contextual Intelligence (CI) in Organisations
Given the rapid evolution of AI, organisations must leverage Contextual Intelligence (CI) effectively. Below are actionable examples of how CI can be implemented in organisations, particularly when dealing with new types of data.
Healthcare Enhancing Patient Care with Predictive Analytics
Acquisition:
Collect diverse datasets, including patient medical histories, real-time health monitoring data from wearables, genetic information, and lifestyle data. This comprehensive data collection helps create a holistic view of each patient. To ensure the accuracy and reliability of the data, employ secure data collection methods such as encrypted electronic health records (EHRs) and secure data transfer protocols. Additionally, integrating data from wearable devices can provide continuous health monitoring, offering valuable insights into patients’ daily activities and physiological changes.
AI Enrichment:
Use AI to analyse these datasets to identify patterns and predict potential health issues. For instance, CI can detect early signs of chronic diseases, allowing for preventive measures. Advanced AI algorithms can process large volumes of data to uncover hidden correlations and trends that might not be immediately apparent to human analysts. By incorporating machine learning models, healthcare providers can enhance the precision of their diagnoses and treatment plans, leading to better patient outcomes.
CI Activation:
Implement personalised treatment plans based on predictive insights. This could involve adjusting medication dosages, recommending lifestyle changes, or scheduling regular checkups. The goal is to provide proactive, rather than reactive, healthcare. Healthcare providers can leverage these insights to tailor interventions specific to each patient’s needs. For example, predictive analytics can help identify patients at high risk for certain conditions, prompting early intervention and potentially preventing the progression of diseases.
Unified Outcome:
By adopting Contextual Intelligence (CI) in healthcare, professionals can achieve a unified outcome of improved patient care and operational efficiency. CI enables healthcare providers to:
- Improve Patient Outcomes: By predicting potential health issues and personalising treatment plans, CI helps in early intervention and better management of chronic diseases.
- Reduce Hospital Readmissions: Predictive analytics can identify patients at risk of readmission, allowing for timely follow-ups and preventive measures.
- Enhance Efficiency: Automated data processing and predictive insights streamline administrative tasks, allowing healthcare professionals to focus more on patient care.
- Ensure Data Security: Implementing robust data security measures protects patient information and ensures compliance with regulations.
Practical Application:
Positioned as “Our healthcare facility will provide you with personalised care and luxury amenities.” Now, wouldn’t you like to come here next time you’re sick?
Retail Optimising Inventory Management with Real-Time Insights
Acquisition:
Gather data from various sources such as sales transactions, customer preferences, seasonal trends, and supplier information. This multifaceted data collection enables a comprehensive understanding of inventory dynamics. Incorporate data from online and offline sales channels, social media sentiment analysis, and market research to obtain a complete picture of consumer behaviour. Utilising advanced data integration techniques ensures seamless data consolidation from disparate sources.
AI Enrichment:
Utilise AI to process and analyse this data, identifying trends and forecasting demand. CI can predict which products will be in high demand during specific periods and which items are likely to become obsolete. Machine learning models can continuously learn from new data, refining their predictions over time. Retailers can also use natural language processing (NLP) to analyse customer reviews and feedback, gaining deeper insights into product performance and consumer preferences.
CI Activation:
Automate inventory management processes based on predictive insights. This includes optimising stock levels, reducing overstock and stockouts, and ensuring timely restocking. Retailers can also personalise marketing strategies to promote slow-moving items or new arrivals. Integrate CI-driven insights with inventory management systems to enable real-time decision-making. Automated reordering systems can ensure optimal stock levels, while dynamic pricing strategies can be employed to maximise sales and profitability.
Unified Outcome:
Through the application of CI, retailers can enhance their inventory management, minimise costs, and improve customer satisfaction by ensuring the availability of desired products.
- Optimise Inventory Management: Predictive analytics helps in maintaining optimal stock levels, reducing overstock and stockouts.
- Personalised Marketing Strategies: Insights from customer behaviour data enable targeted marketing, improving customer engagement and loyalty.
- Improve Supply Chain Efficiency: Real-time data and predictive insights streamline supply chain operations, reducing delays and costs.
- Enhance Customer Experience: Ensuring product availability and personalised recommendations improve the overall shopping experience for customers.
Practical Application:
In South Africa, a few FMCG companies have implemented similar measures or at least partially implemented them. The availability of items at retail stores is irregular in many markets and locations. Wouldn’t it be nice if there was stock available?
Implementing CI Solutions: Tools, Skills, and Costs
Tools and Technologies
To implement CI solutions, organisations need a range of tools and technologies. Data collection tools like Electronic Health Records (EHR) systems, wearables, IoT devices, and CRM systems gather and store data from various sources, providing a comprehensive view of customer behaviours and preferences. Data integration and processing tools such as ETL tools (Talend, Apache Nifi), data warehouses (Amazon Redshift, Google BigQuery), and data lakes (Apache Hadoop, AWS S3) consolidate and process data for comprehensive analysis and high-speed querying. AI and machine learning platforms like TensorFlow, PyTorch, Azure Machine Learning, and AWS SageMaker are used to develop and deploy AI models for tasks like image recognition and natural language processing.
Predictive analytics and business intelligence (BI) tools, including Power BI, Tableau, and Looker, create interactive visualisations and dashboards to turn data into actionable insights. Data security tools, such as encryption software (VeraCrypt, BitLocker), secure transfer protocols (HTTPS, SFTP), and compliance management tools, protect sensitive data and ensure compliance with data protection regulations.
Skills Required
Data scientists and analysts need proficiency in data analysis (R, Python, SQL) and machine learning expertise to analyse datasets and develop predictive models. AI specialists must have skills in designing and fine-tuning AI models and natural language processing (NLP) to solve business problems and enhance efficiency. Data engineers require skills in data integration and database management to set up ETL processes, manage data infrastructure, and ensure data quality.
Cost and Scope
Initial setup costs involve investments in data collection tools, such as procuring and implementing EHR systems, wearables, and CRM systems, as well as AI platforms and infrastructure like setting up AI platforms, cloud services, and data storage solutions. Ongoing operational costs include maintenance of AI models through continuous updates and retraining, data integration and processing costs for ETL processes and data storage, and staff training and development to keep staff updated with new technologies.
Continuous education through training programs and certifications for employees in data science and AI, as well as workshops and seminars, helps keep the team current with industry trends. Scalability considerations involve ensuring that data infrastructure can handle increasing volumes and complexity and building scalable AI solutions that can adapt to growing datasets and business needs.
Conclusion Towards Possibilities
By leveraging CI, organisations across various industries can achieve a unified outcome of enhanced efficiency, better decision-making, and improved customer satisfaction. The integration of AI and human expertise creates a powerful synergy, driving innovation and competitive advantage.