How A Semantic Layer Can Unify AI & BI
A semantic layer can help organisations bridge the gap between BI users and data science teams In the past, business analysts emphasised historical analysis while data science teams in an organisation attempted to surface exciting insights about the future. Business users make better decisions if they anticipate the future. Similarly, data scientists tend to build […]
Topics
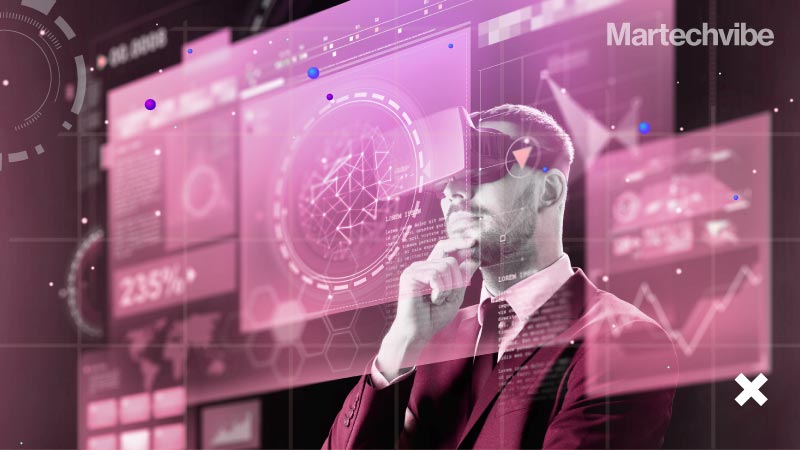
A semantic layer can help organisations bridge the gap between BI users and data science teams
In the past, business analysts emphasised historical analysis while data science teams in an organisation attempted to surface exciting insights about the future. Business users make better decisions if they anticipate the future.
Similarly, data scientists tend to build better models if they compare their predictions to what happened. Historical and predictive analysis are relevant to both teams, but potential interaction is rare.
Today, with the emergence of next-generation infrastructures like the semantic layer, these two silos seem to be coming together. Enterprises that merge these disciplines can deliver best-in-class augmented analytics, aiding the organisation to better understand the past to predict the future.
Importance Of Semantic Layer
A semantic layer represents data that makes it easier for end-users to access data using standards, especially business-friendly terms. The semantic layer maps complex data relationships into easy-to-understand business terms to deliver a unified, consolidated view of data across the organisation.
With a semantic layer, organisations can bridge the gap between BI users and data science teams. This enables teams to work transparently and cooperatively with the same information and goals.
It abstracts away the complexity of underlying raw data for a business model, allowing the consumer of data to access quantitative metrics, attributes, features, predictions, business hierarchies, and complex calculations in an intuitive, easy-to-understand interface.
A semantic layer solution presents a consumer-friendly interface in the “language” of choice, i.e., SQL, MDX, DAX, JDBC, ODBC, REST, or Python, translating several queries into the dialect of the underlying cloud platform. With a standard set of business terms, both teams can interact with the same data, with the same governance rules and results, using the required tools of choice.
With both teams working on the same semantic layer solution, it enables data scientists to easily share or publish their generated features and predictions with business analysts, while business analysts provide feedback to data science teams on the quality of their predictions and changes to be made for tackling model drift.
Once the data becomes highly accessible, teams can collaborate and blend data from second and third-party data sources to unlock the power of data and analytics.
Closing the gap between business intelligence and data science teams is the key to achieving a high level of data analytics maturity and applying all types of analytics at scale.
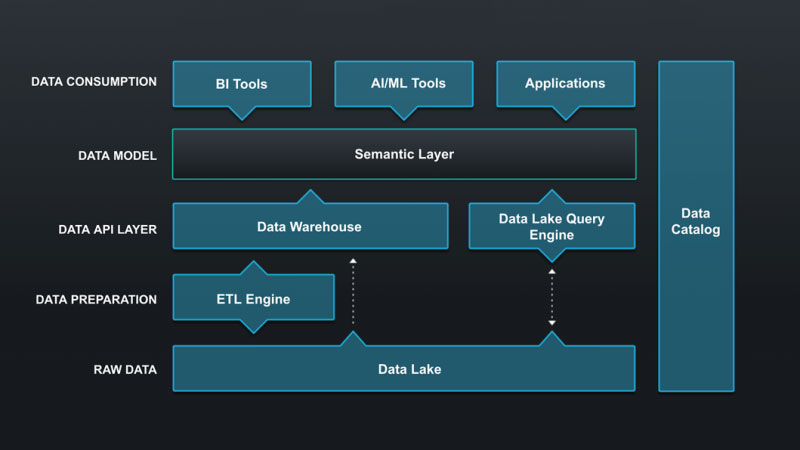
Image Source: Microsoft
Benefits Of Using A Semantic Layer
Establishing a semantic layer can provide several benefits:
- Security: It ensures that data is consistent regardless of consumption style and ensures everyone plays by the same governance.
- Usability: Semantic layer frees business users and data scientists from the dependency on IT and data experts by making data easy to use.
- Agility: Leverages data virtualisation to enable new data landing in data warehouses to be query-able by the BI tool immediately, regardless of its size.
- Performance: By automatically creating and managing aggregates or materialised views inside an underlying data platform, it earns from user query patterns and optimises its performance and cost without data movement.
Better Insights Through AI-BI Unification
When business intelligence and data science teams collaborate using a semantic layer, they can enhance their historical data with better predictive insights. Bridging the gap between business intelligence and data science teams provides more visibility into the output of data science initiatives throughout the organisation. It enables them to leverage their data for predictive and prescriptive analytics.
It enables the creation of augmented intelligence, also known as augmented analytics or decision intelligence, an architecture that brings AI-generated insights into traditional business intelligence workflows to improve data-driven decisions.
When talking about augmented intelligence, most people think of specific features that may appear in AI-enhanced BI tools. Augmenting data through BI and data science unification adds AI-enhanced data to the semantic layer, providing the same insights across the whole spectrum, regardless of the tool used.
A semantic layer helps amplify the effect of the data science teams by sharing their work with a broader audience and providing them with the ability to deliver feedback on the quality and utility of their predictions. Augmented intelligence can transform businesses into data-driven organisations. It starts with implementing the appropriate processes and tools to democratise data and empower individuals to utilise data through self-service analytics.
A semantic layer can act as the driving force for delivering augmented intelligence to a wider audience by working as a medium for publishing the results of data science programs through existing BI channels.
An organisation can capture benefits beyond just historical analysis by feeding data science model results into the semantic layer. It aids decision-makers in consuming predictive insights alongside the historical data.
The same governed data can be used to “drill down” into the details of a prediction for unseen insights. As a result, an organisation can foster more self-service and greater data science literacy and generate a better return on data science investments.
If you liked reading this, you might like our other stories
The Next Generation of Contextual Targeting
Marketing With Data Lakes and Data Warehouses